Opening up a new world of machine learning for biomedical students

“Some of our students don’t even know how to install the program at the start of the course, but by the end they’re programming their own machine learning project to an outstanding level”, says Dr. Bo Wang proudly as he reflects on the first iteration of his new course.
Wang is a joint tenure-track Assistant Professor in the departments of Laboratory Medicine and Pathobiology and Computer Science at the University of Toronto and is an expert in machine learning in medicine. He launched one of the first ever machine learning graduate courses in a medical faculty last Fall.
Basic Principles of Machine Learning in Biomedical Research is a graduate course open to MSc and PhD students at the University of Toronto. Attracting students from departments such as LMP, Molecular Genetics and Medical Biophysics, they don’t need any background knowledge in machine learning, but they “pick it up fast”, says Wang.
What is crucial to Wang is how much hands-on time students get. Around half the course is theory and the rest is doing their own programming and coding. “It’s great to let the students actually do the hands-on work. This lets them discover a real interest in programming which they might not have had a chance to discover otherwise,” he explains.
Students complete a group project by submitting a proposal which Wang reviews and gives them one-on-one advice. The groups then present their project and submit a written report which is peer-reviewed by the rest of the class. “I find the peer review approach very useful. It makes the students relate it to their own work, helping them improve and it exposes them to the scientific review process.”
Running this course for the first time and having students with little or no background, Wang set his expectations low for the projects. “To my surprise many of the projects were of an incredibly high quality, some were even publishable – I was very impressed with them. Many sought to address their own research questions using this new tool they had learned.”
Wang finds the progress students make very rewarding. Many struggle at the start, but by the end they are comfortable using Python and machine learning becomes a tool for them rather than an abstract concept. “It gives students experience and techniques and lets them explore this area and realise that they can do it through their own programming. This helps us train future clinicians and scientists who will understand how to use AI and machine learning in their work because they understand how it can be applied. This path of growth makes me very proud – as well as helping medical students improve their math skills,” he laughs.
The course has been very well received with high enrollments he hopes to expand the course in the future to benefit undergraduate students.
Graduate students at the University of Toronto can enroll in the next course, starting in the Winter Term, through Acorn.
- LMP1210H: Basic Principles of Machine Learning in Biomedical Research
- LMP research in Artificial Intelligence in healthcare
- Q&A with Bo Wang: why collaboration is essential for the future of AI in healthcare
The student voice
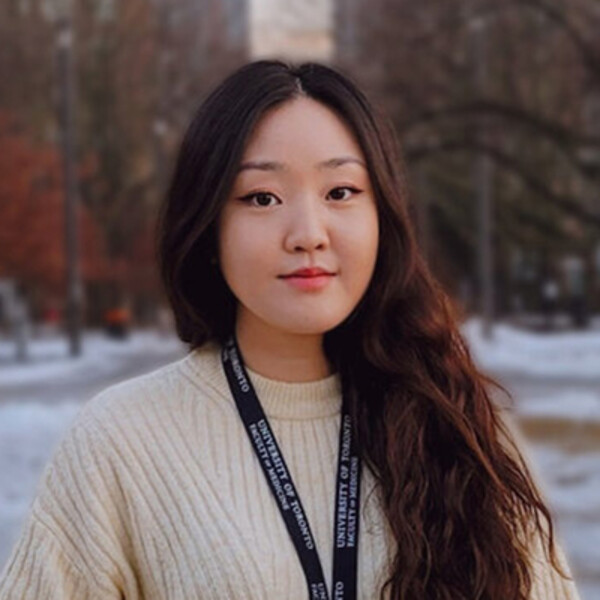
Ain Kim, PhD Candidate in the Department of Laboratory Medicine and Pathobiology
“We produce a lot of data and with this, we can connect a lot of dots that help us understand more about the neurodegenerative diseases we are studying. However, making these connections and manually analyzing the data that we produce is a lot of work that can also introduce a certain level of bias. I joined this course in the hopes that I could incorporate machine learning in my project to improve the efficiency and quality of data analysis as machine learning can help us make sense of the data in innovative ways.
Machine learning courses that are available were more suited for students in computer science. However, I found this course to be tailored to life science students who had no background in computer science, which encouraged me to enroll.
I loved that I learned something that I can actually implement in my own research project. As a person who is far from being tech savvy, learning to code and understanding why my code was or was not working was a big challenge. Understanding the concepts and the math behind machine learning algorithms also took a lot of practice.
I thought that incorporating machine learning in healthcare would be relatively straight forward - gathering data, training the model and getting a predicted outcome. But after putting together our own group project, I realized it was more sophisticated than that. Methods for collecting data vary across different institutions, pre-processing data also depends on who is pre-processing them and what method we use, and most importantly, ethical issues regarding data collection and diagnosis is what we have to work around. With growing number of machine learning models being introduced into healthcare, I wanted to put in more of my time in machine learning research and development.
With the tools that I gained from this course and with the data that I have, I am excited to take my project a step further and integrate machine learning models in healthcare, not necessarily to replace the tools we have but to assist in parallel.”
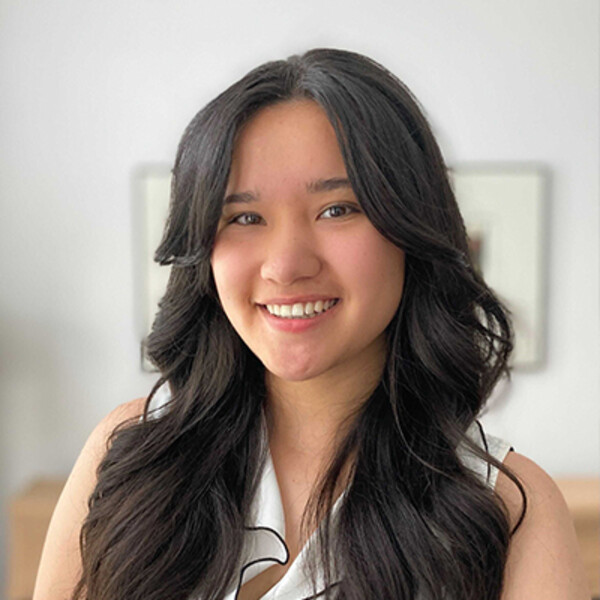
Emily So, Masters of Science in the Department of Medical Biophysics
“I joined this course because I already work in research involving Machine Learning and I was interested in supplementing my education in a healthcare perspective.
This course was different to other grad courses I have taken based on the learning experience. It was much more hands on than other courses and felt less like a “speaker series” - this course offered a lot more translatable skills to tasks.
The highlight for me was how immersive it was. The assignments and projects provided by Dr. Wang prepared you very well to tackle machine learning problems by yourself.
The most challenging part was that the concepts were a bit more advanced in theory than other “Intro to Machine Learning” courses I have taken. Without the previous background in computer science I had, I would have found these concepts to be at a bit steeper learning curve.
It reinforced how machine learning could be applied in very interesting ways to solve well known problems in the clinical space. Now, I am encouraged to try new approaches that I learned from this course for machine learning tasks in my own research.”

Azadeh Bojmehrani, The Translational Research Program (MHSc), Temerty Medicine
“I joined this course because I am so interested in AI and machine learning in medicine - as the technology advances it will improve the health and medical system. This course had such a different and multidisciplinary approach which appealed to me.
The course was easy to follow, focused on specific subjects and had great Teaching Assistant (Adamo Young) and professor. It really challenged me in how we can implement machine learning in medicine.
Now I know more about machine learning and how we can use it to improve healthcare, so I will look more closely to issues and find the unmet need to make new helpful and reliable translational impacts.”
Find out more about the Translational Research Program (TRP)