Two LMP students selected for T-CAIREM Trainee Rounds
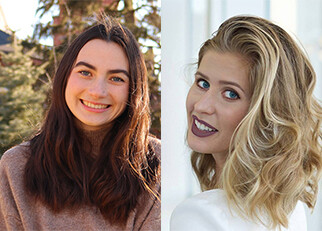
Dominic Ali
Two LMP students, Tara Upshaw and Anglin Dent, were among the 10 researchers selected to participate in the Temerty Centre for AI Research and Education in Medicine (T-CAIREM) 2021 Student Trainee Rounds.
The inaugural competitive seminar series is intended to highlight innovative research at the intersection of artificial intelligence (AI) and healthcare across the University of Toronto’s graduate and professional programs. Students applied by submitting abstracts of their research projects that were judged by a panel of experts.
The 10 selected winners will take part in bi-monthly virtual presentations before leaders in the AI in medicine community. At the conclusion of the seminar series, an expert panel will judge the presented work and select one student to receive the T-CAIREM 2021 Trainee Rounds Research Award valued at $500. The remaining speakers will each receive a $100.
Tara Upshaw: applications, opportunities and challenges for artificial intelligence in primary care
Later this year, Tara Upshaw will deliver her presentation on “Priority applications, opportunities and challenges for artificial intelligence in primary care: Results of a national deliberative dialogue.” Tara will graduate from LMP's MHSc in Translational Research program (TRP) in June and was supervised by Dr. Andrew Pinto in the Department of Family and Community Medicine.
With large volumes of rich data in electronic medical records, primary health care is primed for disruption by artificial intelligence (AI) technology.
Because AI applications in primary care are still limited, there is a unique opportunity to engage key stakeholders in defining how it is used to enhance the discipline.
For her Master’s Capstone research project, conducted in partnership with a social interventions research group called the Upstream Lab at St. Michael’s Hospital, Tara conducted 12 virtual deliberative dialogues with 22 primary care service users, 21 interprofessional providers, and 5 health system leaders from 7 Canadian provinces. Participants identified shared priorities for applying AI in primary care and a number of approaches to navigating anticipated challenges to health AI adoption.
“My research topic arose from the convergence of my fascination with the potential for AI to generate value from the ever-expanding wealth of health data, and a concern about the notable absence of patient and provider voices in guiding the development of health AI technologies, particularly those for use in primary health care”, explains Tara.
“I am honoured to be part of the inaugural cohort of T-CAIREM Student Trainees!”, she adds, “It is a true privilege to be a part of the T-CAIREM network and to learn from my fellow Trainees and others in the University of Toronto community undertaking uniquely inspiring research at the intersections of artificial intelligence and health."
Anglin Dent: using unsupervised deep learning digital pathology tools in tumour morphology
Anglin Dent will present on “Automated resolution of spatial transitions in tumour morphology using unsupervised deep learning digital pathology tools” at a later date. Dent’s supervisor is Dr. Phedias Diamandis.
Anglin first collaborated with the Diamandis AI team as an undergraduate volunteer and was immediately inspired by their research examining how to best integrate AI tools into healthcare.
Having continued working with the lab as a graduate student, she has helped develop tools that aim to replicate the pathology workflow in an automated and efficient way.
“When considering how AI tools may realistically be integrated into the field of pathology and healthcare in general, the development of this workflow is significant, explains Anglin, “The widespread adoption of many developed AI tools has often been limited by time-consuming manual training and rigid parameters that do not adequately mimic the human clinician’s versatility and ability to draw inferences. By leveraging the human-like ability of AI to extract knowledge and discover underlying patterns within data, we have successfully developed a generalizable workflow that can be applied to challenges across cancer sub-classifications.”
“I am very excited for the opportunity to share the development and potential applications of this tool at the T-CAIREM Student Trainee Rounds. I believe unsupervised deep learning techniques could play a key role in the integration of innovative AI tools into clinical practice –increasing efficiency and precision, and ultimately improving patient outcomes.”
More on the T-CAIREM Trainee Rounds
See the T-CAIREM website for a full list of the Trainee Rounds winners and their abstract titles.
Register to attend the first of the Trainee Rounds presentations on April 13, 12 - 1 pm
- Tahera Yesmin: PhD candidate, Department of Mechanical and Industrial Engineering on "A machine learning approach to predict the number of beds that will require cleaning and staff requirements in the emergency department"
- Sujay Nagaraj: MD/PhD candidate, Department of Computer Science and Temerty Faculty of Medicine on "Development and implementation of a machine learning tool to automate vascular catheter access detection in a pediatric critical care unit"