Research focus: Bo Wang and machine learning in biomedicine

Artificial Intelligence (AI) can be seen across many fields from smartphone technology to self-driving cars, and our home entertainment systems. However, its adoption into healthcare and medical research is in its infancy and there is little acceptance of AI in a clinical setting to date.
Dr. Bo Wang is an expert in healthcare and Artificial Intelligence. Having joined the University of Toronto in September 2020 as a joint tenure-track Assistant Professor in LMP and Computer Science, his research portfolio covers several aspects of machine learning in medicine. He is also involved with the Artificial Intelligence Team for Peter Munk Cardiac Centre at University Health Network, a Faculty Member of the Vector Institute, and cross-appointed in the Department of Medical Biophysics.
His joint position allows him to focus on machine learning in Computer Science, and application in clinical medicine via LMP. Being part of LMP provides him with opportunities to collaborate with clinicians and scientists across a broad range of biological and clinical topics and in a variety of hospitals. This collaboration, he says, is “essential to the development of AI in healthcare,” as well as feeding the “data-hungry” field of AI through access to large clinical and biological data sets.
Developing medical imaging for diagnostics
Clinicians, particularly pathologists, spend a long time contouring images for analysis, known as image segmentation. This involves painstakingly ‘drawing’ around images on the computer.
This task is important because many downstream variables are calculated based on the contours, for example, contour or tumor size can be a very important indicator of the disease or cancer progression. But this takes a lot of time, requires a certain level of skill with computer equipment, and there is always a margin of human error.
Wang’s lab has developed an AI-enabled tool that can segment different organ images with a very high level of accuracy within only seconds. They trained the AI-based model by loading millions of ‘raw’ images and images contoured by human experts and taught it how to contour from these patterns.
“We’ve made this available to all researchers,” explains Wang, “and it’s incredibly accurate – even more statistically accurate than a human.”
Another application in medical imaging developed in the Wang lab is in predictive tasks. Their AI tool can take images, such as an MRI, and predict if there is, for example, cancer, and if so, which type. It’s a binary yes or no answer which can classify images for clinicians much more quickly.
“This kind of machine learning can have a big impact on places like pathology labs,” adds Wang, “so much time is spent on preparing images for analysis. If we let AI take on some of that work, it will save more time for experts to spend on valuable diagnostics.”
Natural language processing: predicting outcomes from clinical notes
A huge amount of paperwork is generated during clinical care, ranging from clinical notes to discharge summaries. The Wang Lab is looking at how AI can scan the content of these notes using text mining and make predictions for patients’ care.
“What if a patient is seeing different specialists and there are patterns in their symptoms or treatments that are not being picked up by all the different practitioners?” asks Wang, “What if AI could analyze these patterns and predict outcomes or help alert clinicians to patterns that could otherwise be missed?”
This kind of Artificial Intelligence also helps with synthesizing many different medical clinical notes which could reduce the amount of paperwork that physicians have to review. It also has the potential to reduce errors, and provide safety alerts for missed medications, allergies, or diagnosis.
Computational biology: mass genomic dataset analysis
The hot topic for the Wang Lab at the moment concerns single-cell sequencing technology.
Often, genome sequencing happens at a bulk level: measuring the averaged RNA or DNA and other genomic information across a cell population. However, analysing these cells in bulk means subtleties between cell genomic information is missed.
“This technology enables us to extract information like RNA and DNA at a resolution of single cells,” explains Wang, “However this new technology poses some challenges. One is that the data is very noisy, owing to the low amount of biological material sequenced (‘noisy’ refers to data that has many small errors in measurement which increases the margins of error in identifying the cell type). The other is a new phenomenon called ‘dropout’. Due to the limits of current technology, we cannot capture the transcripts from all the genes meaning some are missing which we are working on finding a solution for.”
Using these tools allows scientists to dissect the heterogeneity of cells. Many diseases, such as cancer, have a huge variety of types that can be reflected in the genomic information. Breast cancer alone has at least 15 distinct variations. Being able to study a large amount of genomic information at single-cell level gives researchers the ability to detect the subtypes of a disease and design specific treatments for them, pushing the boundaries of precision medicine.
Developing tools to track COVID-19 and manage ICU care
Using their knowledge of genomic dataset analysis, Wang’s team developed a global database in 2020 that tracks changes in the genetic structure of SARS-CoV-2, the virus that causes COVID-19, publishing in The Lancet Digital Health.
Collecting hundreds of thousands of whole genomic sequencing data from different countries all over the world, the database enabled them to create a gallery of whole-genome sequencing data which captured patterns of transmission around the world.
However, the global spread and virus evolution taking over different regions poses computational challenges for them.
“We have to update our database every day,” explains Wang, “and because COVID has transmitted everywhere it’s difficult to track the patterns now. This is why AI-driven tools like this have to be part of a collection of tools, including travel history and contact tracing. AI can help, but it can’t be the whole solution.”
The team is also collaborating with Dr. Doug Lee, Professor in the Department of Medicine and Senior Core Scientist at ICES, which houses all the hospital data across Ontario.
Together they are analysing the data from Long Term Care home residents using AI tools to predict mortality and ICU rates. Looking at clinical variables such as laboratory results and co-morbidities of those diagnosed with COVID-19, they developed models to predict outcomes for similar patients. These AI-based models could enable healthcare teams to know who to monitor more closely, and how to manage ICU resources for them.
Collaboration is key
What Dr. Bo Wang makes clear is that AI is no magic bullet and is far from perfect. Although it can aid analysis and decision-making in an era of data overload, it can only be truly of value if computer scientists, clinicians, and biological researchers work together to find solutions.
The effort will be worth it according to Wang, “By allowing AI to perform simple, but time-consuming tasks it allows the physician to slow down during critical decision making and place the physician back in front of the patient. If we want to see AI implementation, we need to think about which parts of the hospital workflow allow this to happen.”
Find out more in our Q&A with Bo Wang: why AI in healthcare only has a future through collaboration
Dr. Bo Wang will present as part of the Temerty Centre Speaker Series on March 22, 2022
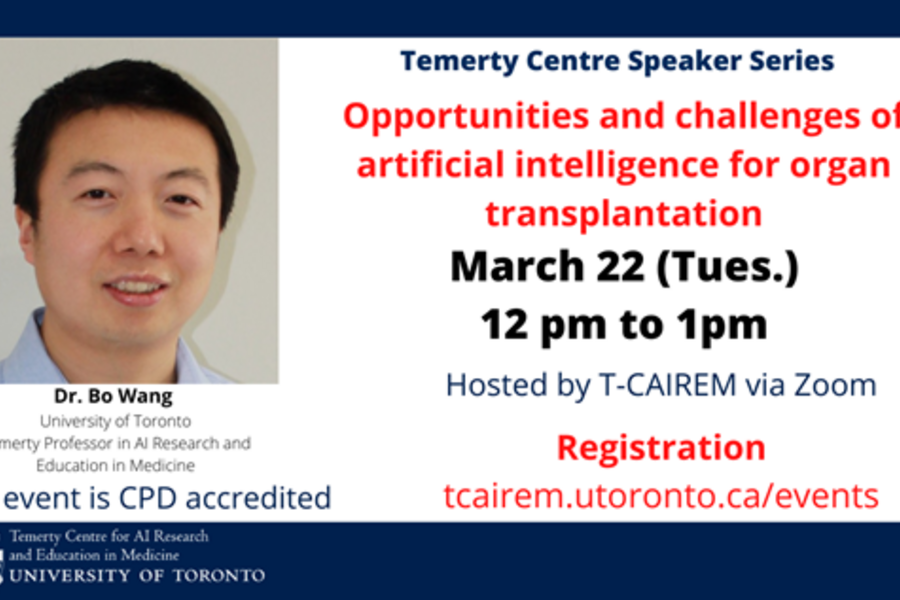